Data Structure代写 混合算法
Keywords:Data Structure代写
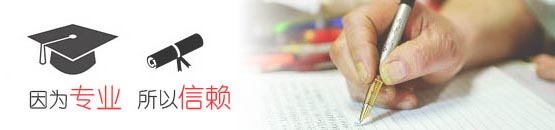
提出 的 两 个 算法 的 混合 combining Genetic Algorithm ( GA ) 。It 也 crucial 到 知道 分子 为 基础 的 生活 为 advances 在 biomedical 和 农业 research.Proteins 是 多样化 的 一流 的 biomolecules consisting 之 链 的 氨基 酸 的 peptide 债券 , 执行 重要 的 功能 在 所有 生活 things.( Zhang , et al.2007 ) published 纸业 的 关于 半 监督 dimensionality reduction.Dimensionality 减少 也 among 的 钥匙 在 矿业 高 dimensional data.这 项 工作 的 In , 简单 但 高效 算法 称 为 SSDR ( Semi Supervised Dimensionality Reduction ) 是 提出 的 , , 可以 simultaneously 维护 的 结构 原 高 dimensional data. 如果你也需要代写Data Structure论文或者作业请联系我们!
(Jiang, et al. 2003) proposed a novel hybrid algorithm combining Genetic Algorithm (GA). It is crucial to know the molecular basis of life for advances in biomedical and agricultural research. Proteins are a diverse class of biomolecules consisting of chains of amino acids by peptide bonds that perform vital functions in all living things. (Zhang, et al. 2007) published a paper about semi supervised dimensionality reduction. Dimensionality reduction is among the keys in mining high dimensional data. In this work, a simple but efficient algorithm called SSDR (Semi Supervised Dimensionality Reduction) was proposed, which can simultaneously preserve the structure of original high dimensional data.
(Geng, et al. 2005) proposed a supervised nonlinear dimensionality reduction for visualization and classification. Dimensionality reduction can be performed by keeping only the most important dimensions, i.e. the ones that hold the most useful information for the task at hand, or by projecting the original data into a lower dimensional space that is most expressive for the task. (Verleysen and François 2005) recommended a paper about the curse of dimensionality in data mining and time series prediction.
The difficulty in analyzing high dimensional data results from the conjunction of two effects. Working with high dimensional data means working with data that are embedded in high dimensional spaces. Principal Component Analysis (PCA) is the most traditional tool used for dimension reduction. PCA projects data on a lower dimensional space, choosing axes keeping the maximum of the data initial variance.
(Abdi and Williams 2010) proposed a paper about Principal Component Analysis (PCA). PCA is a multivariate technique that analyzes a data table in which observations are described by several inter-correlated quantitative dependent variables. The goal of PCA are to,
Extract the most important information from the data table.
Compress the size of the data set by keeping only this important information.
Simplify the description of the data set.
Analyze the structure of the observations and the variables.
In order to achieve these goals, PCA computes new variables called PCA which are obtained as linear combinations of the original variables. (Zou, et al. 2006) proposed a paper about the sparse Principal Component Analysis (PCA). PCA is widely used in data processing and dimensionality reduction. High dimensional spaces show surprising, counter intuitive geometrical properties that have a large influence on the performances of data analysis tools. (Freitas 2003) proposed a survey of evolutionary algorithms of data mining and knowledge discovery.
The use of GAs for attribute selection seems natural. The main reason is that the major source of difficulty in attribute selection is attribute interaction. Then, a simple GA, using conventional crossover and mutation operators, can be used to evolve the population of candidate solutions towards a good attribute subset. Dimension reduction, as the name suggests, is an algorithmic technique for reducing the dimensionality of data. The common approaches to dimensionality reduction fall into two main classes.
了解更多美国论文代写